
How modern organizations prevent million-dollar data disruptions through real-time monitoring
Data in Motion Series, Part 2: Real-Time Pipeline Monitoring Best Practices and ROI
This article is the second of three in our “Data in Motion” series.
Understanding data in motion is crucial. But why, exactly? Ultimately it comes down to your bottom line –– you can prevent business disruption as data flows through your systems. Modern data pipelines span multiple technologies, teams, and transformation stages. Each of those represent potential points of business failure.
The Not-So-Hidden Cost of Data Pipeline Failure
When data pipelines fail, the impact ripples far beyond technical teams. For instance, Southwest Airlines December 2022 scheduling system failure, triggered by data synchronization issues in the crew scheduling system, led to over 16,700 cancelled flights. It cost the airline $800 million in lost revenue and compensation.
Similarly, Knight Capital Group's 2012 trading incident, caused by incorrect data deployment across servers, resulted in $440 million in losses in just 45 minutes.
Rapid data flow issues can quickly cascade into severe business impacts. What’s more, they’re also a PR nightmare for organizations.
Monitoring Data in Motion at Every Stage
From pipeline to production, preventing data management pitfalls happens at each step of the data lifecycle. Monitoring data in motion is an important component. Here’s what that looks like in practice.
Ingestion
When data first gets moving, ingestion monitoring acts as the first line of defense. Stream processing validation ensures data completeness before downstream systems engage. Schema evolution detection watches for breaking changes in moving data structures. System health monitoring identifies upstream issues before they cascade through streaming pipelines. All of these functions can be performed with an operational observability solution in place.
Storage
Even in storage, data remains in constant motion through replication and backup streams. Real-time quality and observability metrics detect anomalies during write operations. And capacity forecasting can prevent pipeline stalls by monitoring data motion volumes.
Transformation
Data in motion faces its greatest test during transformation. Lineage tracking maps how data changes as it flows through processing steps. Real-time performance monitoring catches early signs of degradation in data movement. Freshness metrics ensure transformations maintain the speed of business operations.
Enrichment
As flowing data combines with other sources, enrichment monitoring is also vital. Integration points track the confluence of data streams, while version control ensures that reference data stays current with moving datasets. Other analysis confirms that enriched data maintains integrity while in motion.
Consumption
In consumption, data in motion reaches its crescendo. Real-time anomaly detection catches issues before user impact. Pattern monitoring tracks how data flows to end users. End-to-end latency monitoring measures total transit time from ingestion to consumption. Cost tracking prevents unexpected resource drain from high-velocity data movement.
Prevention Success Stories
Robust monitoring has prevented catastrophic consequences. We’ve seen above some of the times when that prevention came too late, or didn’t come at all. Here are some examples of just-in-time prevention, where data in motion monitoring delivered a major ROI.
Netflix
Netflix’s data pipeline processes over 1.3 trillion events per day through their real-time monitoring and analytics tools. Their stream processing architecture allows early detection of playback issues and content delivery problems before they impact viewer experience at scale.
Target
In 2021, Target's investment in data systems and digital reliability drove a 20.8% growth in digital comparable sales, meaning their data pipeline monitoring enabled more scalable digital operations.
TQL
Total Quality Logistics, North America's second-largest freight brokerage firm, implemented pipeline monitoring and data observability to address reliability issues in their business intelligence infrastructure. Through improved data pipeline visibility and monitoring, they reduced their BI reports from over 3,000 to under 500 while maintaining business capabilities.
Data in Motion Monitoring: Types of ROI
Monitoring data as it flows through systems delivers measurable returns across multiple business dimensions. Financial returns come from preventing costly outages and maintaining service continuity. Operational gains emerge through faster issue detection and reduced time-to-resolution. Customer satisfaction improves when problems are caught before impacting service delivery.
Most importantly, data in motion monitoring helps your team shift from a reactive to a proactive organization. Instead of discovering issues through customer complaints or system failures, teams catch and resolve problems during transit. It’s about opportunity cost; data issues that would otherwise slow down your business momentum – don’t.
Data in Motion Continued…
In our next piece, we'll explore a critical yet often overlooked aspect of data in motion: pipeline execution monitoring. We'll dive into how failed job initiations can silently derail data operations, and how modern tools and predictive analytics are changing the game for data reliability.
Read Data in Motion Part 1 here
Read Data in Motion Part 3 (Coming Soon)
Keep Reading

November 4, 2024
Why Data Observability is Essential for Generative AI (GenAI)Discover the real-world benefits and how companies can optimize GenAI applications by implementing robust data observability practices.
Read More
October 10, 2024
Why Most Data Observability Platforms Fall ShortIn boardrooms across the globe, executives are grappling with a painful truth: the data tools they've invested in aren't delivering on their promises. Learn why in this blog.
Read More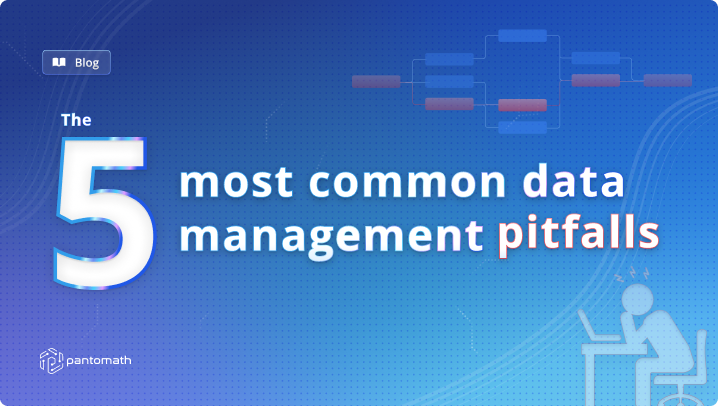
September 18, 2024
The 5 Most Common Data Management PitfallsThere are five common mistakes that teams often make on their journey toward data observability. Learn how to avoid them!
Read More