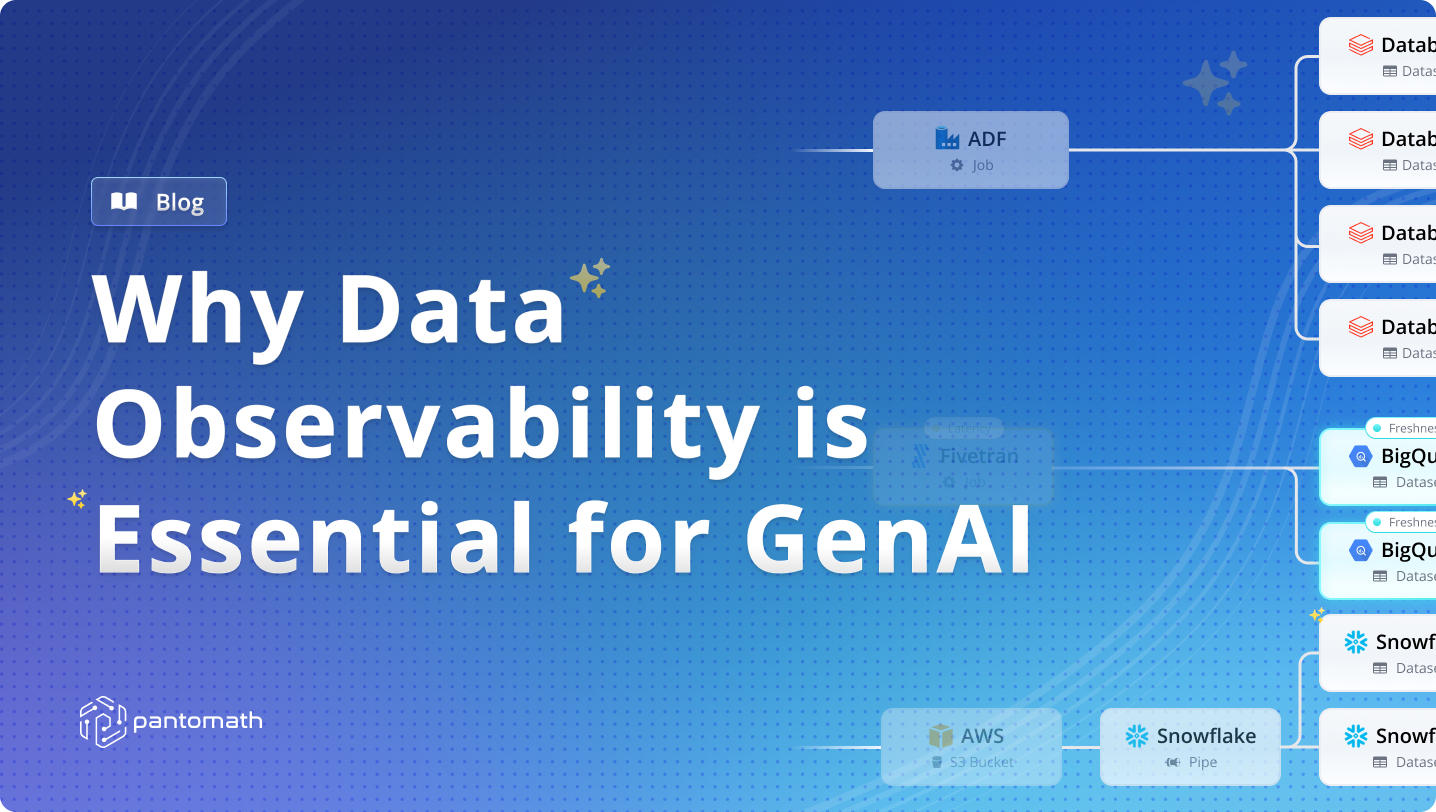
Discover the real-world benefits and how companies can optimize GenAI applications by implementing robust data observability practices.
Why Data Observability is Essential for Generative AI (GenAI)
In the race to adopt generative AI, organizations are discovering an unexpected bottleneck: data quality. According to Forrester's recent research, 70% of enterprise companies are already using generative AI. Another 20% are actively exploring its implementation. Yet, in all of the buzz, data quality emerges as the primary factor limiting successful genAI deployments.
That might seem counterintuitive at first glance. LLMs are trained on vast amounts of unstructured data from the internet. Can’t they handle a bit of imperfect data? The reality is more complex, and the stakes are high.
Traditional data quality approaches were designed for the world of structured databases and predictable queries. GenAI has shattered these assumptions. These systems consume data at unprecedented speed and scale. They make intuitive leaps across data sources that traditional data management teams could never have predicted. And, when an AI model generates an inaccurate response or makes a misleading recommendation, the impact can cascade through your business instantly, before traditional data quality mechanisms catch it.
Consider this: while traditional data monitoring might surface a contained error in a pipeline, genAI can use that same incorrect data to generate customer communications, make business recommendations, or inform critical decisions – all with the confidence and authority that makes these systems so powerful.
GenAI systems don't just query databases – they make creative connections across vast amounts of data, generate new content, and make decisions at machine speed. And unlike traditional data monitoring, with nothing but confidence and bravado, AI can make the wrong decision thousands of times per second.
The old adage "garbage in, garbage out" has therefore taken on new meaning. GenAI brings new business risks and potential data management pitfalls.
That’s where data observability becomes critical. Not as a nice-to-have monitoring tool, but as the fundamental foundation that makes enterprise genAI possible. Modern data observability goes beyond simple quality checks and monitoring. It provides the comprehensive visibility that keeps systems trustworthy for your organization.
In this post, we'll explore the unique challenges of genAI, why data observability is essential for AI, and the real-world benefits of data observability in genAI.
Unique data challenges of genAI
To understand why traditional approaches fall short, let's first compare how data challenges differ between traditional applications and genAI systems:
Speed and scale issues
The velocity and volume of data processing in genAI applications dwarf traditional data workflows. Consider these operational realities:
- A customer-facing genAI chatbot might need to process thousands of concurrent requests, each requiring access to multiple data sources within milliseconds
- Training data pipelines to implement data pipeline automation may require massive volumes of both structured and unstructured data
- Real-time inference requires immediate access to up-to-date information across various business domains
The unpredictability factor
GenAI systems tend to make unexpected connections across data sources. Traditional data pipelines are predictable: you know exactly which tables and fields will be queried and how they'll be used.
With genAI, when a user asks a question, the system might need to:
- Access historical customer interactions from multiple databases
- Pull relevant product documentation
- Reference current pricing and inventory data
- Incorporate recent policy updates
- Cross-reference with compliance guidelines
That happens dynamically, which makes it impossible to predict which data sources will be needed at any given moment.
Data issue amplification
The impact of data quality problems can be magnified for genAI. A single incorrect data point might be used to generate hundreds of AI responses. Outdated information can be confidently presented as current fact. Inconsistencies across data sources can lead to contradictory AI outputs. And, training data issues might not be apparent until the model is already in production. When it’s a question of magnitude, genAI can increase the magnitude and scale of an issue.
Data observability solves genAI’s biggest problems
It prevents costly failures
The cost of genAI failures extends far beyond immediate financial impact – it can damage brand reputation and bring AI initiatives to a screeching halt.
Take customer service AI as one example. Without observability, a chatbot providing incorrect refund policies might interact with hundreds of customers before an issue is detected. With proper observability, these issues are caught within minutes, potentially saving hundreds or thousands of dollars per incident in cleanup costs and lost customer goodwill.
It ensures AI model performance
Data observability ensures AI model performance by addressing two critical areas: training data quality and data drift detection. There are a few critical types of drift that can impact model performance. Early detection of these issues prevents model degradation and maintains accuracy throughout the AI system's lifecycle.
It enables trust
People don’t trust genAI systems yet; and with good reason. That’s why it’s all the more important to create a sense of transparency and reliability where you can. Data observability provides visibility into AI decisions, through features like data lineage tracking and end-to-end pipeline traceability. Teams can trace any response back to source data, so they can rest assured that they’re not working with hallucinations.
It reduces resolution times
Perhaps the most immediate benefit of data observability is the dramatic reduction in incident resolution time. Here's how:
Modern data observability platforms can:
- Automatically detect anomalies before they impact users
- Provide detailed context for faster troubleshooting
- Suggest remediation steps based on historical patterns
- Track resolution progress and effectiveness
A real-world example of data observability in action
Paycor, a leading HCM software provider serving over 30,000 customers, faced challenges with their complex billing operations that required reviewing data within a tight 24-hour window at month-end.
After implementing Pantomath, they transformed their ability to detect and resolve data issues, moving from reactive end-of-month troubleshooting to proactive detection weeks before issues could impact invoices. The solution provides end-to-end visibility across four data platforms in Paycor's ecosystem, enabling the team to detect failed jobs, missing data, and other issues early while significantly reducing the time needed to identify root causes and implement fixes, ultimately improving both operational efficiency and customer satisfaction.
Real-world benefits of data observability in genAI
Customer service
Data observability transforms customer-facing AI applications by monitoring the entire interaction pipeline. When a customer interacts with an AI system, it needs to pull from multiple data sources including customer history, product information, and policy documents — all of which must be reliable and up-to-date. As demonstrated in the Paycor example, moving from reactive to proactive monitoring allows teams to spot potential issues weeks in advance rather than discovering problems through customer complaints.
Content generation
For AI content generation, data observability provides crucial monitoring of both input and output data streams, helping prevent hallucinations. Teams can verify that AI-generated content is grounded in accurate data through lineage. Data observability prevents the generation of plausible but incorrect content.
Business intelligence
In business intelligence applications, data observability ensures that genAI systems maintain reliable insights for decision-making by monitoring data freshness and quality across all sources. That means teams arrive at AI-generated business insights and support in much faster timeframes.
Final thoughts
Implement data observability now, or implement it later, but you’ll want to implement it as you integrate generative AI into your business model. It will be your life raft, when you need to validate and trace data in real-time. Trust, compliance, and innovation follow.
The future belongs to organizations who can harness the power of genAI, without letting it spiral out of control within the data ecosystem. In this landscape, data observability emerges as the essential bridge between AI's potential and its practical, reliable implementation in the real world.
Ready to walk through data observability for genAI in your organization? Schedule a demo with Pantomath today.
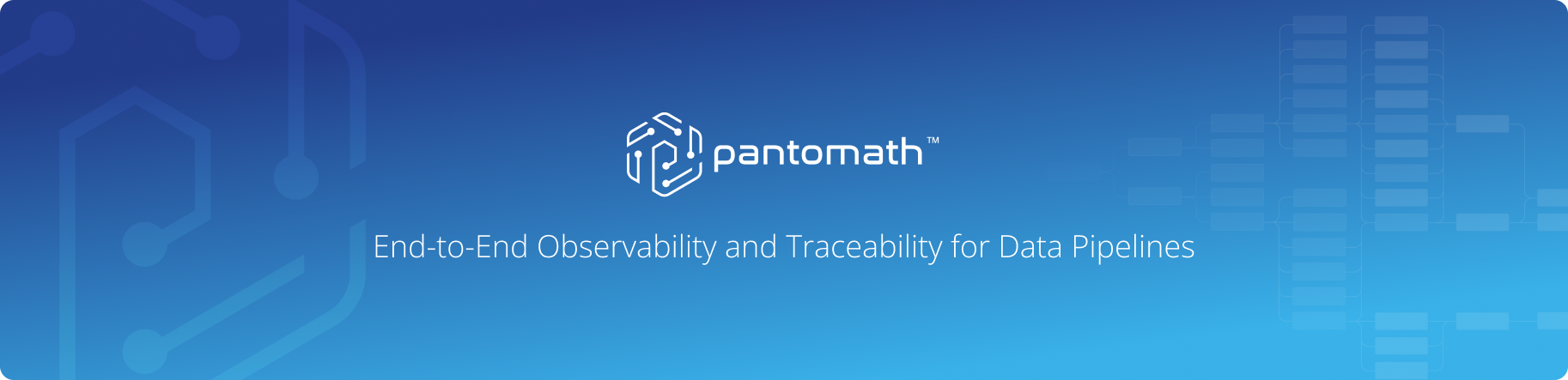
Keep Reading

October 10, 2024
Why Most Data Observability Platforms Fall ShortIn boardrooms across the globe, executives are grappling with a painful truth: the data tools they've invested in aren't delivering on their promises. Learn why in this blog.
Read More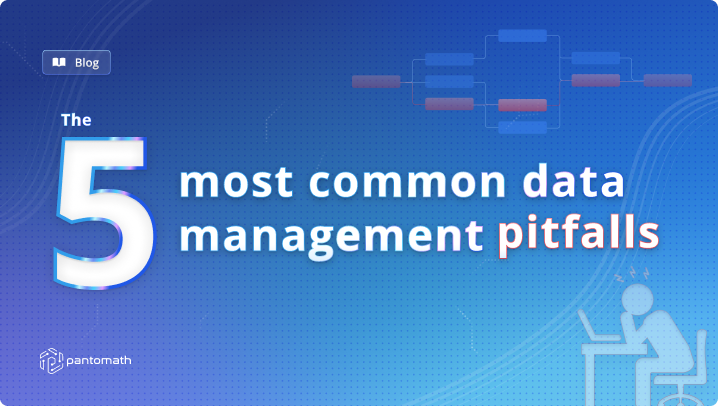
September 18, 2024
The 5 Most Common Data Management PitfallsThere are five common mistakes that teams often make on their journey toward data observability. Learn how to avoid them!
Read More
August 7, 2024
10 Essential Metrics for Effective Data ObservabilityYou can’t simply implement data observability and then hope for the best. Learn about the top 10 essential metrics to make your business thrive.
Read More