
Data quality and reliability is paramount to the success of modern-day organizations. Data quality issues can lead to significant business disruptions, poor decision-making with bad data, and overall lack of trust in data. Learn about Pantomath's unique approach to data quality.
The Pantomath Data Quality Framework
Data quality and reliability is paramount to the success of modern-day organizations. Data quality issues can lead to significant business disruptions, poor decision-making with bad data, and overall lack of trust in data. Our customers have observed that 90% of data quality issues are driven by leaky data pipelines. These issues are caught with operational & data observability by highlighting issues with your dataset at a macro level when jobs fail, have issues with inconsistent scheduled start times, or run long which typically result in freshness or volume issues in datasets. But what do you do about the other 10% of data quality issues?
The Challenge of Data Quality
Data quality issues are often difficult to identify, meaning data consumers, or those impacted by the data quality issue, are the first to recognize and report them. This leads to the data engineering team playing defense, always reacting to data quality issues, and hunting down the problem, rather than focusing on delivering value and new analytics to the business.
Modern tools and solutions for data quality do a decent job recognizing data quality issues reactively, but they unfortunately struggle with enabling actions to remediate the issue – what was the root cause, how to fix the issue, what was the downstream impact and who to notify. They also require data level access to be granted to a third-party vendor, often having unknown cost implications due to robust queries running in your warehouse and are a standalone check without context of your data pipelines end-to-end.
Our Framework
Data quality checks are best when they exist embedded within data pipelines. This allows the flexibility to leverage native data quality solutions like Snowflake Horizon, Databricks Lakehouse Monitoring, Google Dataplex or write complex custom checks directly in line with your data in any data platform.
The Pantomath Data Quality Framework is built on our foundation of traceability and operational observability. Data quality checks are seamlessly integrated with Pantomath like any other asset and auto recognized as a data quality check. Through our traceability framework, the datasets being checked, and the downstream impact can be easily understood.
When these data quality checks fail within the platform of record, Pantomath flags these data quality checks as a Data Quality incident within our incident management framework. This provides users with a clear line of sight to see where these data quality incidents have impacted downstream processes and data consumers, and how ‘bad’ data has propagated through the pipeline.

Make Data Quality Actionable
Data quality should be about action. Data engineers are responsible for providing reliable data to the organization, not simply providing transparency into bad data.
Data quality incidents in Pantomath allow users to quickly understand what went wrong, how to resolve the issue and what is impacted downstream. By developing data quality checks directly in a data platform, our Data Quality Framework provides the capability to offer contextual messages as a result of the failure of a data quality check directly in Pantomath. Pipeline embedded checks can deliver detailed insights into the nature and cause of data quality incidents, as well as exactly what data quality issues are being flagged.
This level of detail is invaluable for troubleshooting and resolving data quality issues quickly. It empowers data engineers to take immediate corrective actions, reducing downtime and ensuring that data pipelines continue to run smoothly and deliver high quality data to inform critical decision making.
Ownership & Security
Embedded data quality checks allow our users unlimited control. Data engineers, who know their data best, can craft specific and complex checks tailored to meet the needs of your data and business requirements.
This control also allows data quality checks to be implemented with a lower cost of ownership. You retain full control over which data is checked, how much of the data is scanned and how often these checks occur. This ensures you are minimizing cost and getting notified only for the data quality checks that matter most, avoiding alert fatigue.
By embedding data quality checks directly within the platform of record, organizations eliminate the risk of vendor lock-in, and our seamless integration ensures that data quality checks are part of existing data pipelines and workflows. Furthermore, these checks are implemented directly by your teams – this means that Pantomath does not require read access to underlying data thus minimizing compliance and security concerns. It also ensures that you have a direct line of sight to the underlying data within tables, reducing the risk of unauthorized access and data breaches since the raw data remains under the user's control without exposure to a third-party vendor.
Conclusion
Data quality checks are best when they are embedded within the data pipeline living directly with the data. They provide unmatched control and customization, maintain a security-first approach by keeping data visibility within the customer's domain, and align with the latest industry trends.
However, data quality checks alone do not solve the problem of data reliability. Pantomath’s Data Quality Framework offers the best of both worlds. Data Quality checks can be created with data, cost, and security top of mind, seamlessly integrated into Pantomath’s end-to-end observability and traceability framework, and actioned like any other data incident.
If you are interested in scaling data quality with seamless integration and best-in-class security, schedule a demo with our expert team. We are excited to share details about our modern and innovative cloud-based solution.
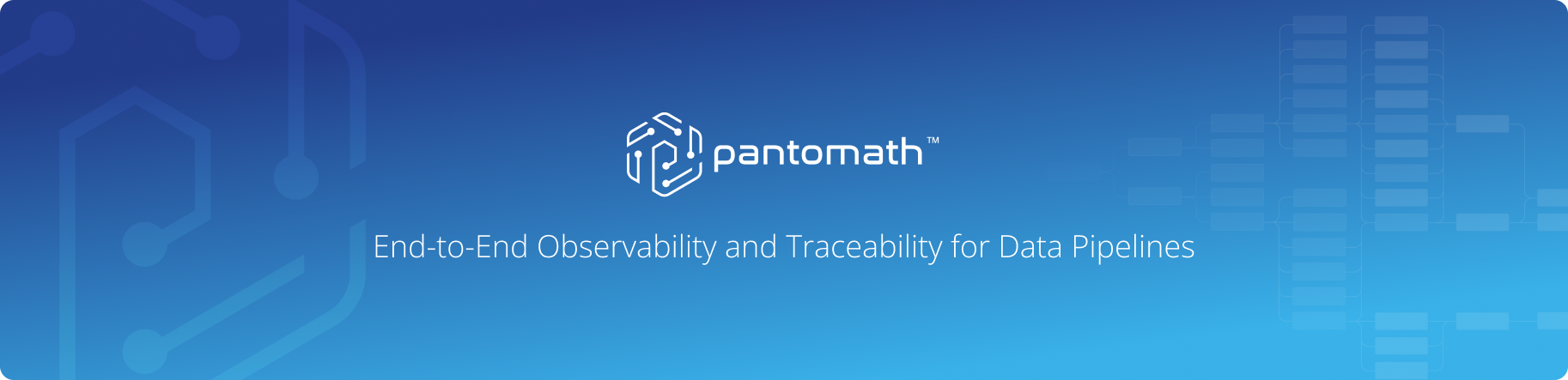
Keep Reading

October 10, 2024
Why Most Data Observability Platforms Fall ShortIn boardrooms across the globe, executives are grappling with a painful truth: the data tools they've invested in aren't delivering on their promises. Learn why in this blog.
Read More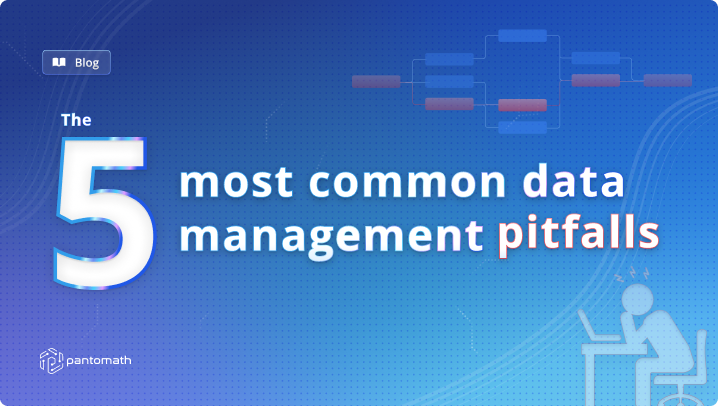
September 18, 2024
The 5 Most Common Data Management PitfallsThere are five common mistakes that teams often make on their journey toward data observability. Learn how to avoid them!
Read More
August 7, 2024
10 Essential Metrics for Effective Data ObservabilityYou can’t simply implement data observability and then hope for the best. Learn about the top 10 essential metrics to make your business thrive.
Read More