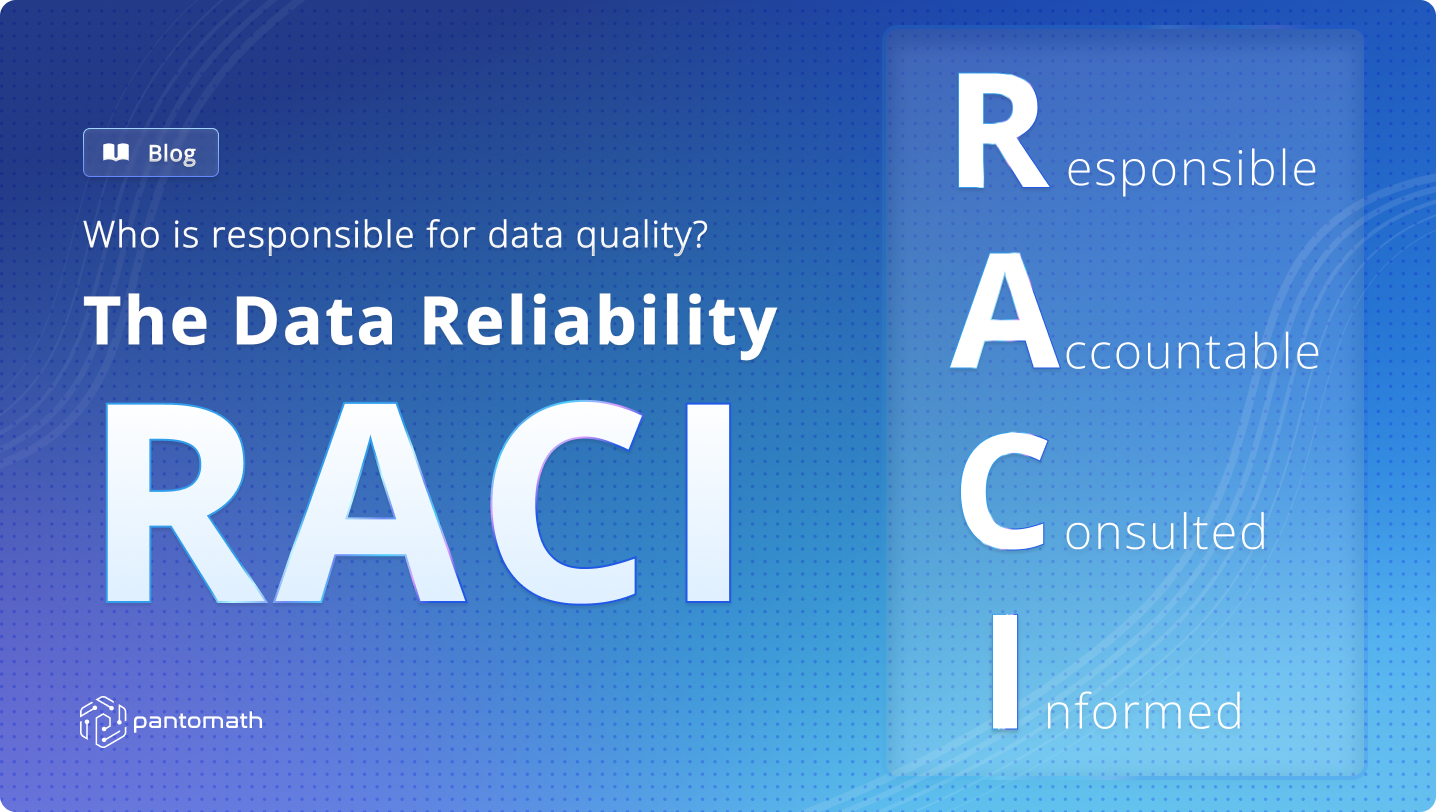
Learn about who should share ownership and responsibility for data quality and reliability in an organization from Craig Smith, Pantomath's VP of Customer Experience.
Data Quality Roles and Responsibilities | The Data Reliability RACI
Who should share ownership and responsibility for data quality and reliability in an organization? In enterprises with distributed data landscapes, diverse datasets, and complex data pipelines, this is a difficult question to answer.
Data engineers are bound to view data pipelines from the lens of each vertical technology in the data stack, however, they lack visibility to how the puzzle pieces fit together. A horizontal and cross-functional comprehension of the data pipeline is needed. Data operations and data platform teams share a more holistic view of the flow of data from ingestion to visualization. They lack the context and understanding of the data pipelines that the data engineer has. Data consumers understand what the data should look like in its end state and its business use however they don’t know how the sausage is made.
No one role can own data quality and reliability for an organization. Ultimately, it’s a team sport. Without clarity on who performs what tasks and how the roles work together, every data quality issue has the potential to disrupt the business and erode confidence in valuable data.
Enterprises can get closer to operationalizing data reliability with consistent workflows for addressing data quality and clearly defined responsibilities for all involved. My combined 30+ years of experience in the data space working with companies like AOL, Netezza, IBM and Alation amongst others, juxtaposed to working with customers like Lendly and Coterie Insurance at Pantomath, a clear RACI matrix for data reliability has emerged.
In this blog, we’ll outline the roles and responsibilities that help enterprises operationalize data reliability.
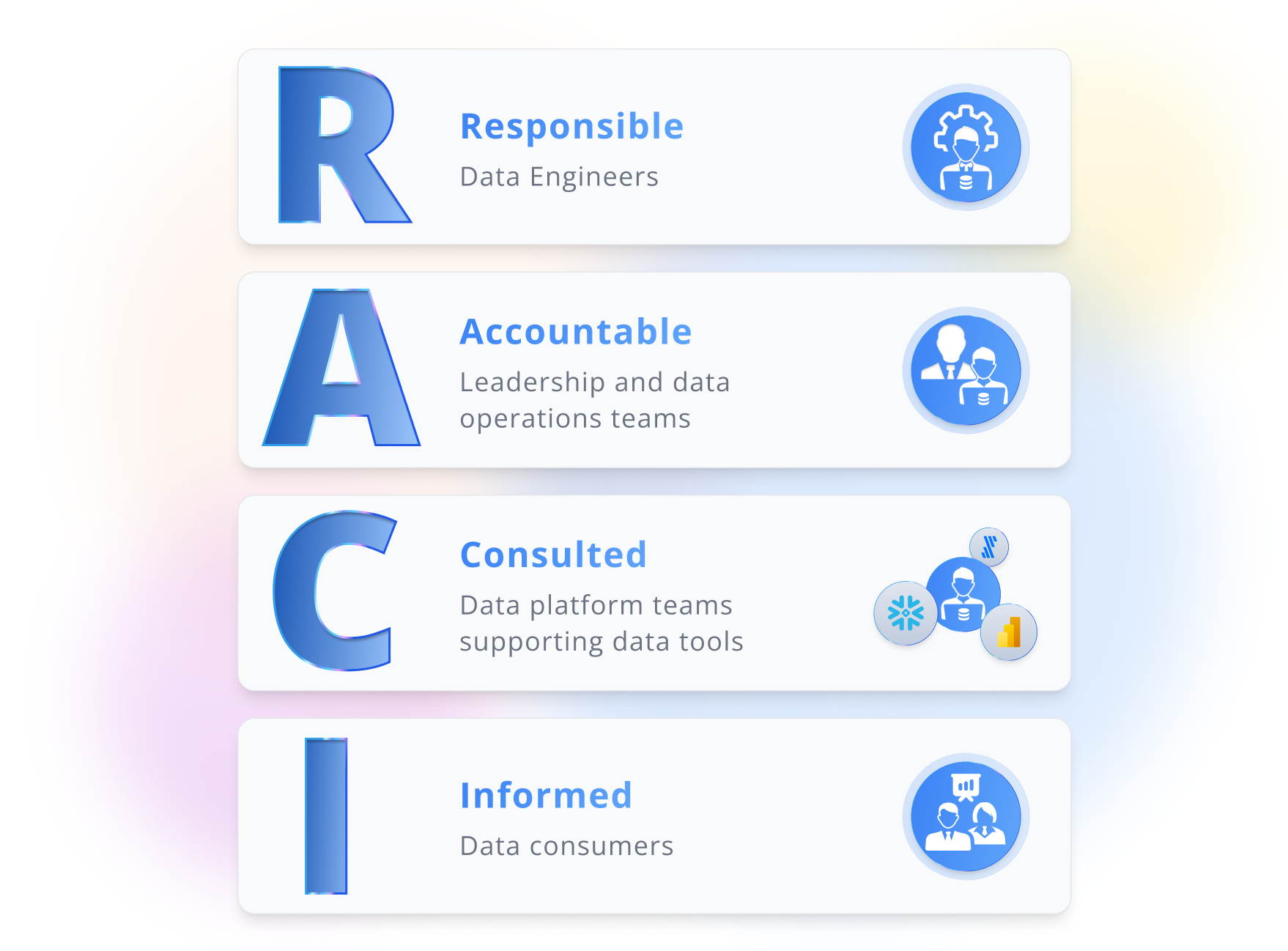
The Data Reliability RACI
Let’s define who is responsible, accountable, consulted, and informed in an enterprise structured to increase data reliability.
Responsible: Ultimately, the data engineers are responsible for resolving data quality issues. They have the context around the data pipeline and understand the affected technologies – whether that’s data integration, data orchestration, data warehousing, etc. They are the ones who can get in and fix the root cause that affects data quality.
Accountable: While data engineers are getting hands-on and fixing issues, leadership and data operations teams are accountable for the reliability of data pipelines. Data operations teams are often the first line of defense for supporting the health of data pipelines and triaging data incidents; these support teams communicate with end users and coordinate with data engineers, ensuring end users are up to date on the status of the incident while data engineers focus on resolution.
Consulted: Data platform teams supporting data tools and their underlying infrastructure are often consulted by the operations teams and data engineers to pinpoint the origin of the issue. These platform teams own the administration of the data tools and technologies that might be involved in the data incident and are intimately familiar with their inner workings as well as subtle nuances. They also have visibility to health of the underlying infrastructure of data tools that can at times be the root cause of data reliability and quality issues.
Informed: Data consumers are informed. They need to be updated on the status of the data pipelines and the health of the data to ensure that the business experiences minimal disruption from data quality issues. This ensures a culture of data transparency and avoids poor decision-making with incorrect data.
The Data Reliability Workflow Without Automated Data Observability
With the roles more clearly defined, let’s look at how they interact when there are data quality issues.
In the first scenario, an L1 support engineer from the data operations team detects a failed job within a platform in the data stack. The failed job might lead to missing or stale data in at least one downstream report. The operations engineer informs an L3 data engineer through the organization’s incident management tool who then gets to work, investigating the failed job and its root cause.
The data engineer also consults the data platform team who then tries to take an inventory of everything downstream of that failed job. They try to determine what else has been impacted across different data pipelines powering reports. Additional L3 support engineers and data engineers whose data pipelines have been impacted get pulled into the fire drill. They in turn notify the end users of impacted reports that there is an issue potentially impacting their ability to make business decisions.
Each team stays in communication through collaboration tools and incident management platforms. They provide timely updates to business stakeholders and in some cases executives, depending on the criticality of the report. The communication continues until the root cause of the issue has been fixed and downstream impact points have been remediated, with up-to-date and refreshed data across all the data pipelines.
In many ways, this is a best-case scenario. Most of the time, it’s end users that identify the problem first, raising the complaint to multiple leaders in the hopes of quick identification and resolution.
Let’s discuss a common scenario: in this case, a data consumer notices that their BI dashboard looks “off”. The data appears out-of-date, and a member of the marketing team raises the issue with the operations team by opening a support case. The marketing team is under pressure to get the data for a forthcoming quarterly review, so this is an urgent scenario.

Under the pressure of this deadline, the operations team connects with several of the data engineers and platform engineers responsible for the data and supporting platforms that feed the BI report. Without any idea of what the root cause might be, they need to retrace the flow of data until they can pinpoint it.
While the appropriate data engineers and platform teams manually reverse engineer the data pipelines across each data platform to ascertain what caused the issue, the operations team provides frequent yet ineffectual updates to the marketing team – they just cannot provide clarity in the moment. It can take hours, even days, to retrace the complex web of pipelines and resolve issues of this nature.
It’s probably obvious from this example that even with the right roles in place and the right chain of communications, data reliability is still difficult to achieve. Data consumers are often the first to encounter data quality issues, which puts data engineers and operations teams in a reactive posture and forces them to respond to acute issues rather than proactively address them. Reverse-engineering complex data pipelines across different platforms in the hope of identifying root cause and understanding the impact of the issue can be incredibly time-consuming and costly, distracting these teams from creating more valuable data products.
Data reliability with end-to-end data observability
End-to-end data observability automates and streamlines the traditional data reliability workflow. Rather than relying on data consumers to be the canary in the coal mine, the data team is automatically alerted to issues before data consumers are affected.

With an end-to-end data observability platform like Pantomath, pipeline traceability removes the need to painstakingly reverse-engineer complex data pipelines. Data teams are automatically alerted to the root cause of issues and their impact in real time. In addition, Pantomath streamlines communication, enabling data engineers to focus on their work and giving operations teams a clear view of the health of the data platform. Without impact on downstream analytics, the need to communicate the status of data quality issues with end users is greatly reduced. This end-to-end data observability drives trust in data, eliminates poor decision-making with bad data and saves productive time for all the teams involved.
If you are interested in operationalizing data reliability, schedule a demo with our expert team. We’re excited to share details about our modern and innovative cloud-based solution.
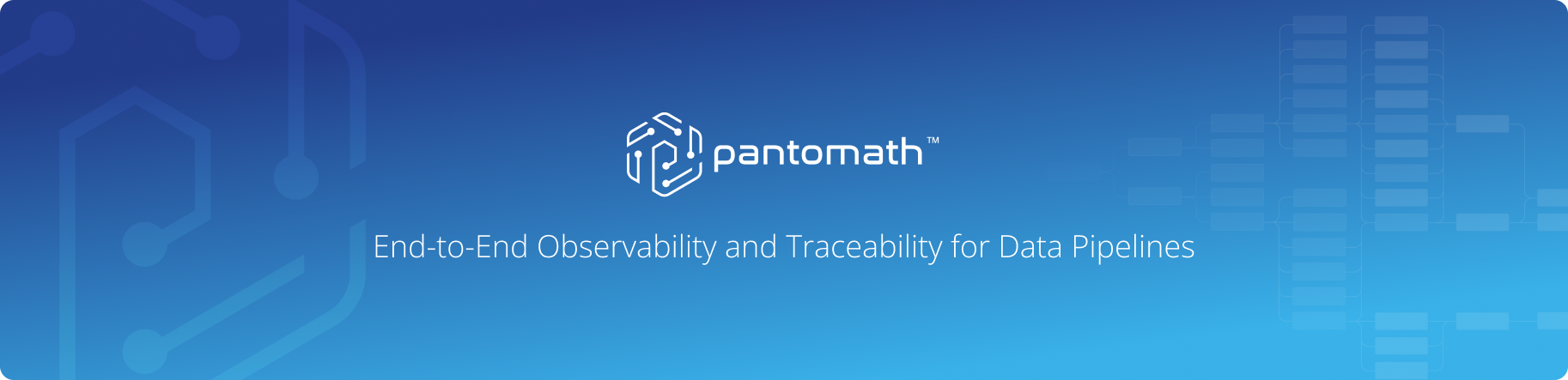
Keep Reading
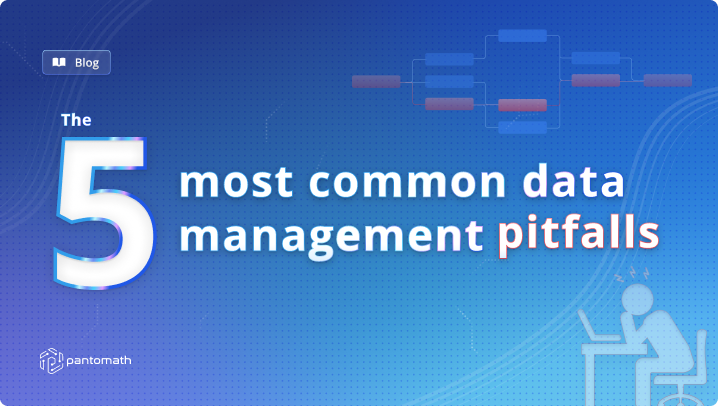
September 18, 2024
The 5 Most Common Data Management PitfallsThere are five common mistakes that teams often make on their journey toward data observability. Learn how to avoid them!
Read More
August 7, 2024
10 Essential Metrics for Effective Data ObservabilityYou can’t simply implement data observability and then hope for the best. Learn about the top 10 essential metrics to make your business thrive.
Read More
July 26, 2024
The Evolution of Data Quality: Why Data Observability is KeyData quality has a long history, dating back at least to mainframes in the 1960s. Learn about the path Data Quality has taken, and where it's headed.
Read More