
Data quality has a long history, dating back at least to mainframes in the 1960s. Learn about the path Data Quality has taken, and where it's headed.
The Evolution of Data Quality: Why Data Observability is Key
Data quality has a long history, dating back at least to mainframes in the 1960s. Modern approaches became prominent with the rise of relational databases and data warehouses in the 1980s. Since then, the approaches to data quality have had to change to keep pace with how we analyze and use data, from business intelligence to big data, machine learning, and artificial intelligence.
In recent years, data quality is always placed near the top of chief data officer (CDO) priorities. And with the meteoric rise of generative AI, data quality is arguably more important than ever. Although our approaches to data quality have changed many times over the years, we need yet another evolution to keep up with the growing demands for data and the complexity of the modern enterprise.
In this blog, we’ll take a look at the evolution of data quality, and examine the next leap needed to meet the needs of the modern enterprise.
The Single Source of Truth
While data quality has deep roots, we’re jumping to the 1980s when data warehousing began to fuel business-driven data analysis. Relational database and data warehousing giants like IBM, Teradata, and Oracle made data more available than it had ever been before for driving business decisions.

During this period, the concept of Master Data Management (MDM) started to emerge. With MDM, data from many different systems is de-duplicated and combined to create a “single source of truth.” In this paradigm, there was one, tightly controlled “golden record” of the data. Data profiling, cleansing, enrichment, and validation processes were used to ensure that the data was accurate, complete, consistent, up-to-date, and adhered to the rules of the business.
Business Intelligence Scratches the Golden Record
In the 1990s, with the lowered cost of data warehousing, the push came to make data even more accessible and to put data in the hands of more business decision-makers – not just top management.
Computers became everyday office tools and extract transform load (ETL) and online analytical processing (OLAP) made it easier to flow data into tools for visualizing data. Business intelligence (BI) became a mandatory tool for enterprises, spurred on by offerings from computing giants like IBM, Microsoft, SAP, and Oracle.

MDM had become the de-facto way to ensure data quality, spurred on by the need to use data across different business lines and the introduction of regulations like the Sarbanes-Oxley Act in 2002.
Despite its adoption, the cracks in MDM were already starting to show. BI and the influx of data created on the internet strained the ability of IT teams to effectively control the flow of data and meet the needs of the business. Webs of data pipelines now needed to be maintained to support the growing sprawl of reports and dashboards built for specific business purposes.
Big Data Breaks the Mold
While the introduction of BI made ensuring data quality more challenging, “big data” broke the mold.
With all the data being created on the internet – much of it unstructured, companies found themselves awash in customer data. Storing data in a single database no longer made sense. As the volume of data grew, companies could often end up with dozens of disconnected databases with different users and purposes.
At the same time, cloud computing lowered the cost of storage with Google BigQuery, and later with AWS Redshift and Snowflake, while the introduction of the “data lake,” first built on the Hadoop framework, enabled the collection and analysis of massive amounts of unstructured data.
Now, the focus switched from maintaining a “golden record” to storing everything and figuring it out later. The sheer volume, variety, and speed at which data was created and the ‘store-everything’ mindset made MDM just about impossible and quickly outpaced specialized tools and tests.
DJ Patil famously called data scientists the “sexiest job” of the 21st century for their ability to “coax treasure out of messy, unstructured data.” The reality was often less than sexy. Data scientists spent as much as eighty percent of their time wrangling data into shape for analysis. And as machine learning became more commonplace, traditional methods of ensuring data quality became obsolete.
Data Observability Attempts to Scale Data Quality
Data observability emerged to bring automation to data quality and to help scale data quality to the modern data stack. Now, the average enterprise has numerous data pipelines to maintain, numerous tools for orchestration and integration, and numerous clouds for storing and computing data. Rather than relying on hand-written rules or Python packages to attempt to address data quality, data observability would continuously monitor the data and apply machine learning to detect anomalies.
While an improvement over the tooling that had come before, data observability only looked at data at rest. It ignored the tangled web of data pipelines and tools that brought the data to the warehouse, data lake, report, or dashboard. This limited view missed key issues, like data latency, data movement, and other problems that occur when data is in motion.
By only looking at data at rest, data observability also doesn’t identify the root cause of data quality and reliability issues. While data observability can alert when something goes wrong, it can do little to help remediate the issue. As a result, data teams must painstakingly reverse-engineer data pipelines to try to figure out what went wrong and how to fix it – a process that can take hours if not days.
Data Pipeline Observability & Traceability Completes the Picture
Today, every enterprise runs on data, and that data is supported by complex, interdependent environments where one issue upstream in the cross-system data pipeline can have a devastating impact on all the downstream functions that rely on it.

To truly address data quality in these complex data environments, organizations need a view into the pipelines that feed their reports, dashboards, algorithms, models, and applications. Cross-platform pipeline traceability completes the goal of scaling data quality that was started with data observability.
Data observability and data pipeline traceability together create end-to-end data observability. With end-to-end data observability, enterprises have a complete view of their data at every stage of the pipeline from the source systems to the reports and at every hop in between.
If you're interested in improving data quality and reliability and want to explore more about end-to-end data observability, arrange a demo with our expert team.
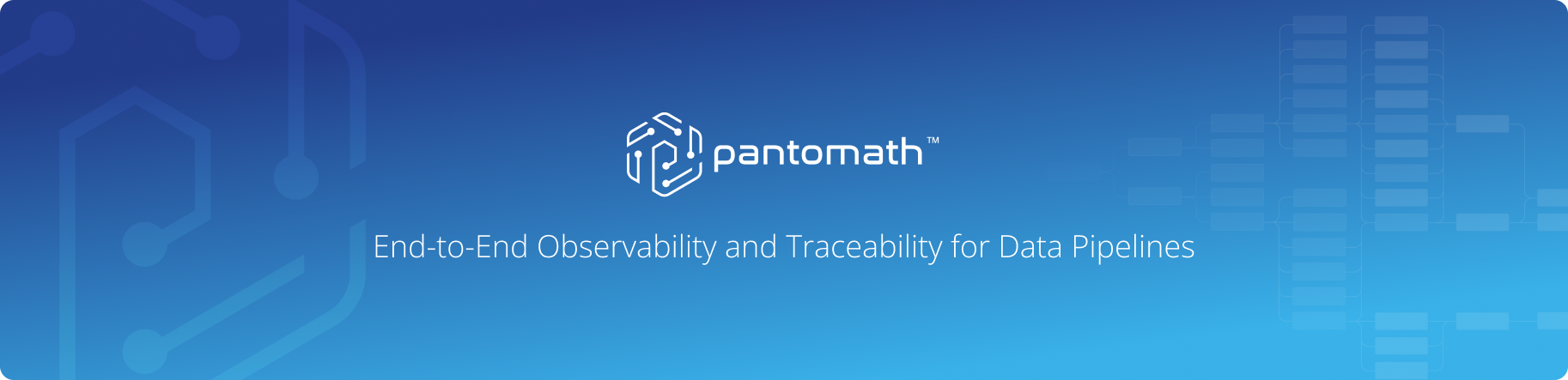
Keep Reading

October 10, 2024
Why Most Data Observability Platforms Fall ShortIn boardrooms across the globe, executives are grappling with a painful truth: the data tools they've invested in aren't delivering on their promises. Learn why in this blog.
Read More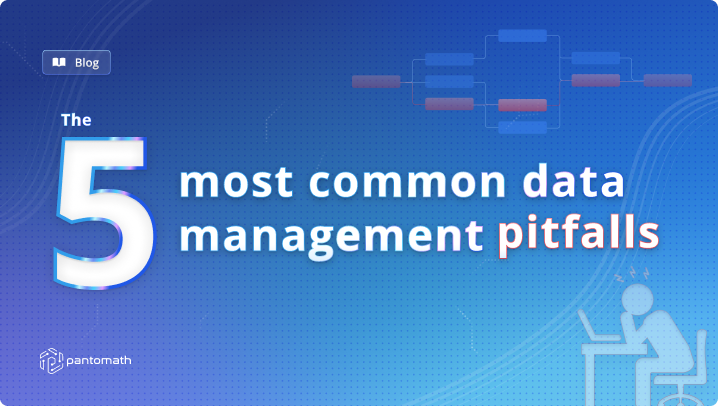
September 18, 2024
The 5 Most Common Data Management PitfallsThere are five common mistakes that teams often make on their journey toward data observability. Learn how to avoid them!
Read More
August 7, 2024
10 Essential Metrics for Effective Data ObservabilityYou can’t simply implement data observability and then hope for the best. Learn about the top 10 essential metrics to make your business thrive.
Read More